Introduction
The potential of large language models (LLMs) to enhance the scientific research process is garnering significant attention. A recent study available on arXiv investigates the use of GPT-4 for generating peer feedback on research manuscripts. By addressing the challenges posed by the rapid growth of scholarly output and specialized knowledge, the study highlights the role of AI for academic research, providing valuable insights into the effectiveness and practical utility of LLM-generated feedback, particularly for junior researchers and those in under-resourced settings.
Having carefully studied the entire research paper, we have distilled its primary insights, presenting them in a straightforward and accessible manner for your benefit.
Methodology
The model used: OpenAI’s GPT-4 was the large language model (LLM) used, whose training data extends only until 2021 and employed datasets from 2022 to avoid bias.
Datasets used: 3,096 accepted papers, 8,745 reviews from 15 Nature family journals, 1,709 papers, and 6,506 reviews from the International Conference on Learning Representations (ICLR), all published between 2022-23.
Prompting strategy: GPT-4 was provided with the main sections of each paper and specific instructions to generate a structured scientific review with four feedback areas in a single step.
Comparison: A system that summarizes and matches comments from GPT -4 and human reviewers was built to measure how much their feedback overlaps. A survey was conducted to determine whether the output is helpful for researchers.
Results
GPT-4-generated feedback significantly overlapped with human reviewers’ comments in both datasets:
Nature Family Journals: 57.55% of GPT-4’s comments matched those of at least one human reviewer in Nature Family Journals.
ICLR: 77.18% of GPT-4’s comments aligned with human feedback in the ICLR dataset:
Open image-20241202-055834.png
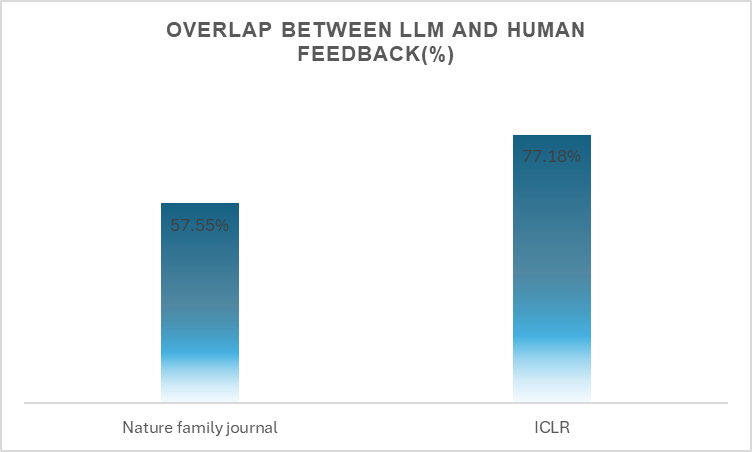
Fig: Graph highlights the percentage overlap between GPT-4-generated feedback and human feedback
Additionally, the overlap between GPT-4 and individual human reviewers was comparable to the overlap observed between two human reviewers themselves.
The survey also highlighted that half of participants found LLM-generated feedback not only helpful in improving their work but, in many cases, as effective—or even better—than human feedback
What this means about using AI for academic research
These results indicate that LLM-generated feedback is almost as reliable and useful as human feedback, particularly in identifying issues in papers that require revisions. The high degree of overlap between LLM and human reviewers demonstrates that LLMs can effectively highlight critical areas needing improvement, ensuring the quality and integrity of the research.
Benefits to the medical community
Researchers – LLM-generated feedback serves as a valuable resource for researchers seeking constructive manuscript enhancements, especially those without access to timely quality feedback mechanisms like conferences and peer reviews.
Students – It will assist students in understanding and improving their research papers, enhancing their academic skills and knowledge.
Institutes – It reduces the need for extensive internal peer review processes by providing an additional layer of feedback, thereby optimizing institutional resources.
Conclusion
In conclusion, the study demonstrates that LLM-generated feedback effectively complements traditional peer review by showing significant overlap with human feedback and receiving positive user evaluations. This underscores the future for AI for academic research, highlighting the potential of platforms like Dx to greatly enhance medical research by providing accessible, high-quality feedback and supporting researchers in advancing their work to a substantial extent.
Read the full research study on arXiv. Additionally, did you know that Dx has a module dedicated to medical research? You can explore it here and let us know if you have any feedback.